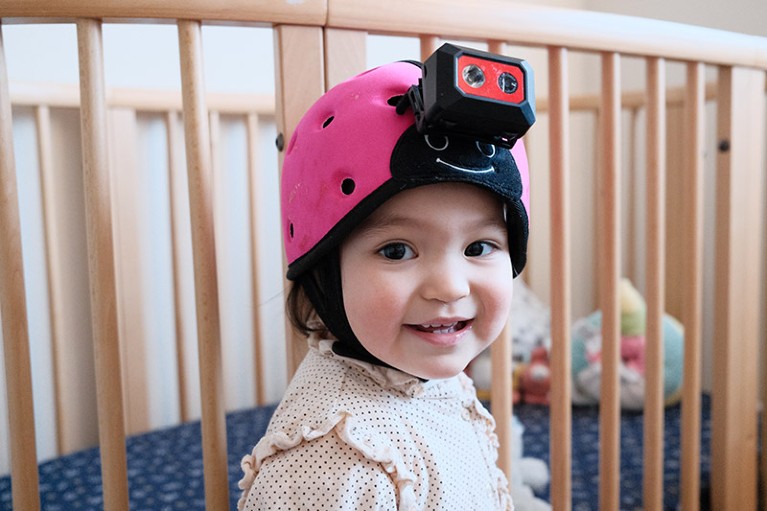
The artificial intelligence learned using video and audio from a helmet-mounted camera worn by Sam — here aged 18 months.Credit: Wai Keen Vong
An artificial intelligence (AI) model has learnt to recognize words such as ‘crib’ and ‘ball’, by studying headcam recordings of a tiny fraction of a single baby’s life.
The results suggest that AI can help us to understand how humans learn, says Wai Keen Vong, co-author of the study and a researcher in AI at New York University. This has previously been unclear, because other language-learning models such as ChatGPT learn on billions of data points, which is not comparable to the real-world experiences of an infant, says Vong. “We don’t get given the internet when we’re born.”
The authors hope that the research, reported in Science on 1 February1, will feed into long-standing debates about how children learn language. The AI learnt only by building associations between the images and words it saw together; it was not programmed with any other prior knowledge about language. That challenges some cognitive-science theories that, to attach meaning to words, babies need some innate knowledge about how language works, says Vong.
The study is “a fascinating approach” to understanding early language acquisition in children, says Heather Bortfeld, a cognitive scientist at the University of California, Merced.
Baby’s-eye view
Table of Contents
Vong and his colleagues used 61 hours of recordings from a camera mounted on a helmet worn by a baby boy named Sam, to gather experiences from the infant’s perspective. Sam, who lives near Adelaide in Australia, wore the camera for around one hour twice each week (roughly 1% of his waking hours), from the age of six months to around two years.
The researchers trained their neural network — an AI inspired by the structure of the brain — on frames from the video and words spoken to Sam, transcribed from the recording. The model was exposed to 250,000 words and corresponding images, captured during activities such as playing, reading and eating. The model used a technique called contrastive learning to learn which images and text tend to go together and which do not, to build up information that can used to predict which images certain words, such as ‘ball’ and ‘bowl’, refer to.
To test the AI, the researchers asked the model to match a word with one of four candidate images, a test that is also used to evaluate children’s language abilities. It successfully classified the object 62% of the time — much better than the 25% expected by chance, and comparable to a similar AI model that was trained on 400 million image–text pairs from outside this data set.
For some words, such as ‘apple’ and ‘dog’, the model was able to correctly identify previously unseen examples — something humans generally find relatively easy. On average, it did so successfully 35% of the time. The AI was better at identifying objects out of context when they occurred frequently in the training data. It was also best at identifying objects that vary little in their appearance, says Vong. Words which can refer to a variety of different items — such as ‘toy’ — were harder to learn.
Lessons about learning
The study’s reliance on data from a single child might raise questions about the generalizability of its findings, because childrens’ experiences and environments vary greatly, says Bortfeld. But the exercise revealed that a lot can be learnt in the infant’s earliest days through only forming associations between different sensory sources, she adds. The findings also challenge scientists — such as US linguist Noam Chomsky — who claim that language is too complex and the input of information is too sparse, for language acquisition to happen through general learning processes. “These are among the strongest data I’ve seen showing that such ‘special’ mechanisms are not necessary,” says Bortfeld.
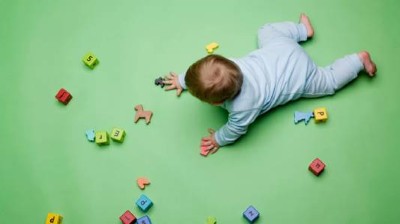
DeepMind AI learns simple physics like a baby
Real-world language learning is much richer and varied than the AI experienced. The researchers say that, because the AI is limited to training on still images and written text, it could not experience interactions that are inherent to a real baby’s life. The AI struggled to learn the word ‘hand’ for example, which is usually learnt early on in an infant’s life, says Vong. “Babies have their own hands, they have a lot of experience with them. That’s definitely a missing component of our model.”
“The potential for further refinements to make the model more aligned with the complexities of human learning is vast, offering exciting avenues for advancements in cognitive sciences,” says Anirudh Goyal, a machine learning scientist at the University of Montreal, Canada.