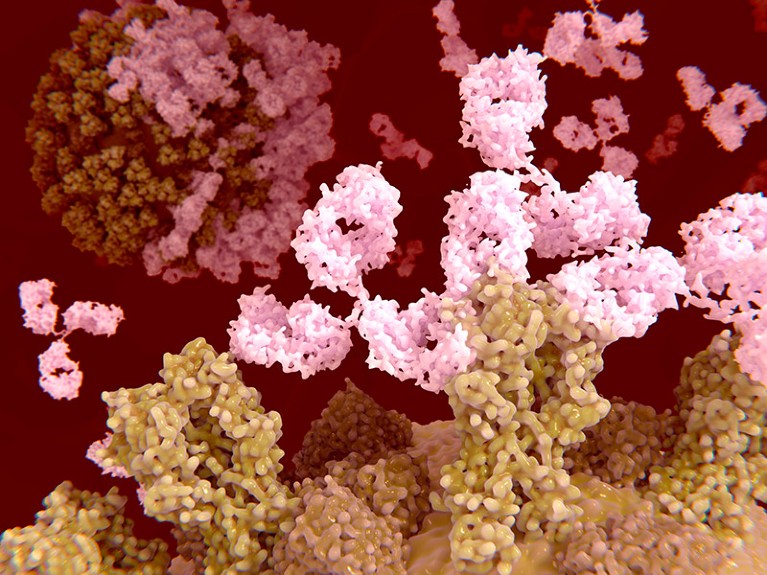
Antibodies (pink) bind to influenza virus proteins (yellow) (artist’s conception).Credit: Juan Gaertner/Science Photo Library
Researchers have used generative artificial intelligence (AI) to help them make completely new antibodies for the first time.
The proof-of-principle work, reported this week in a preprint on bioRxiv1, raises the possibility of bringing AI-guided protein design to the therapeutic antibody market, which is worth hundreds of billions of dollars.
AlphaFold touted as next big thing for drug discovery — but is it?
Antibodies — immune molecules that strongly attach to proteins implicated in disease — have conventionally been made using brute-force approaches that involve immunizing animals or screening vast numbers of molecules.
AI tools that can shortcut those costly efforts have the potential to “democratize the ability to design antibodies”, says study co-author Nathaniel Bennett, a computational biochemist at the University of Washington in Seattle. “Ten years from now, this is how we’re going to be designing antibodies.”
“It’s a really promising piece of research” that represents an important step in applying AI protein-design tools to making new antibodies, says Charlotte Deane, an immuno-informatician at the University of Oxford, UK.
Making mini proteins
Table of Contents
Bennett and his colleagues used an AI tool that their team released last year2 that has helped to transform protein design. The tool, called RFdiffusion, allows researchers to design mini proteins that can strongly attach to another protein of choice. But these custom proteins bear no resemblance to antibodies, which recognize their targets by way of floppy loops that have proved difficult to model with AI.
To overcome this, a team co-led by computational biophysicist David Baker and computational biochemist Joseph Watson, both at the University of Washington, modified RFdiffusion. The tool is based on a neural network similar to those used by image-generating AIs such as Midjourney and DALL·E. The team fine-tuned the network by training it on thousands of experimentally determined structures of antibodies attached to their targets, as well as real-world examples of other antibody-like interactions.
How generative AI is building better antibodies
Using this approach, the researchers designed thousands of antibodies that recognize specific regions of several bacterial and viral proteins — including those that the SARS-CoV-2 and influenza viruses use to invade cells — and a cancer drug target. They then made a subset of their designs in the laboratory and tested whether the molecules could bind to the right targets.
Watson says that about one in 100 antibody designs worked as hoped — a lower success rate than the team now achieves with other types of AI-designed protein. The researchers determined the structure of one of the influenza antibodies, using a technique called cryo-electron microscopy, and found that it recognized the intended portion of the target protein.
Early proof of principle
A handful of companies are already using generative AI to help develop antibody drugs. Baker and Watson’s team hopes that RFdiffusion can help to tackle drug targets that have proved challenging, such as G-protein coupled receptors — membrane proteins that help to control a cell’s responses to external chemicals.
But the antibodies that RFdiffusion churned out are a long way from reaching the clinic. The designer antibodies that did work didn’t bind to their targets particularly strongly. Any antibody used therapeutically would also need its sequences modified to resemble natural human antibodies so as not to provoke an immune reaction.
The designs are also what’s known as single-domain antibodies, which resemble those found in camels and sharks, rather than the more complex proteins that nearly all approved antibody drugs are based on. These types of antibody are easier to design and simpler to study in the lab, and it makes sense to design these first, says Deane. “But this doesn’t take away from it being a step on the way to the kinds of methods we need.”
“This is proof-of-principle work,” Watson stresses. But he hopes this initial success will pave the way for designing antibody drugs at touch of a button. “It feels like quite a landmark moment. It really shows this is possible.”